A practical to guide to identifying and preventing tall tales by AI
What are AI Hallucinations?
06 January 2025
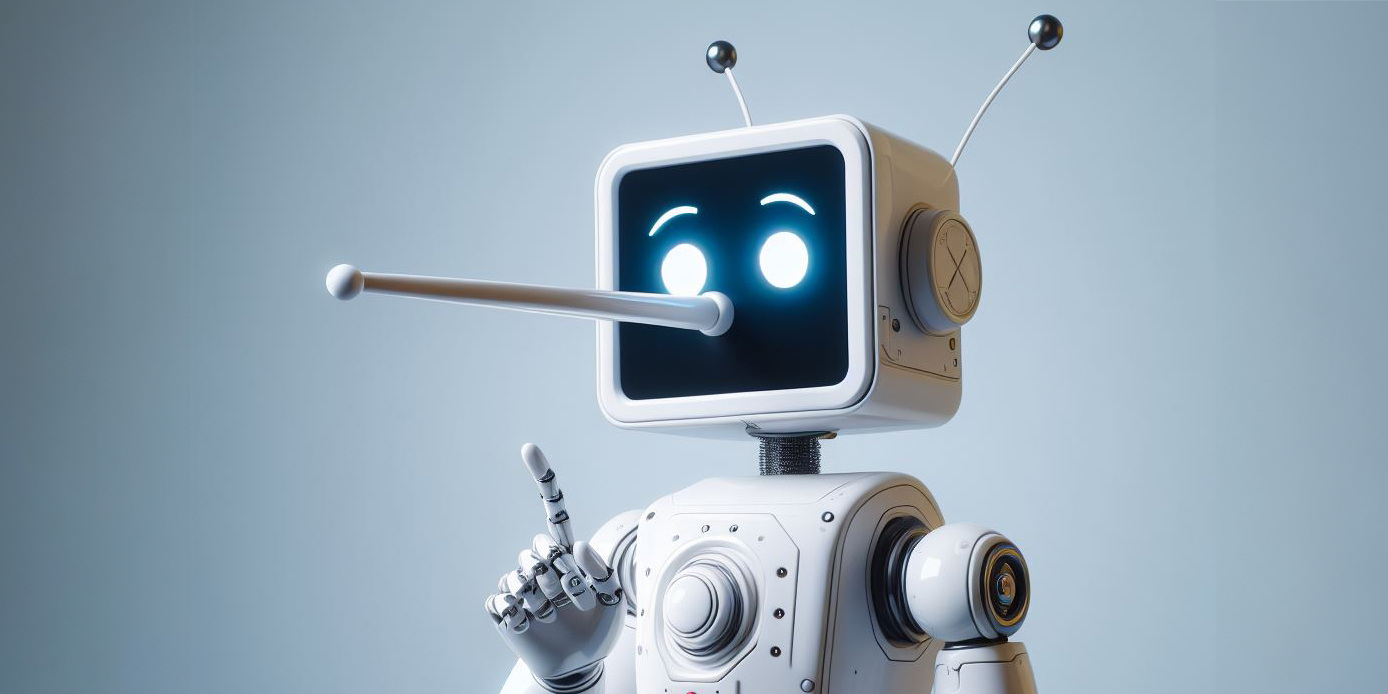
AI hallucinations are AI-generated outputs that are factually incorrect, nonsensical, or inconsistent, due to bad training data or misidentified patterns.
01
What are AI hallucinations?
On the hour and day that we checked (because, as you know, the information changes all the time), here's how various authoritative resources summarize AI hallucinations:
Merriam-Webster on AI hallucinations
According to Merriam-Webster, a computing hallucination is a plausible but false or misleading response generated by an artificial intelligence algorithm. It bases this definition on a quote from an interview with Prabhakar Raghavan appearing in Germany's Welt am Sonntag newspaper, stating that artificial intelligence can sometimes lead to an hallucination, "expressed in such a way that a machine delivers a convincing but completely fictitious answer."
Bing on AI hallucinations
According to Microsoft Bing, AI hallucinations refer to:
- Phenomena where a large language model perceives patterns or objects that are nonexistent or imperceptible to human observers, creating nonsensical or inaccurate outputs.1
- Responses generated by AI that contain false or misleading information presented as fact.2
- Incorrect or misleading results generated by AI models due to factors like insufficient training data, incorrect assumptions, or biases.3
- Inaccurate information produced by generative AI models that appears confident but can be harmful.4
Google AI overview on AI hallucinations
According to Google's AI overview, AI hallucinations occur when an artificial intelligence (AI) system generates incorrect or misleading information:1 | 2
-
Explanation3
AI hallucinations can occur when AI models perceive patterns or objects that aren't real or are difficult for humans to see. This can lead to AI models producing outputs that are inaccurate or nonsensical. -
Causes4
AI hallucinations can be caused by a variety of factors, including:
– Insufficient training data
– Incorrect assumptions made by the model
– Biases in the data used to train the model
– The model being given the wrong tasks -
Examples5|6
AI hallucinations can range from comically fake to slightly off base. For example, an AI might respond to a query about a person's accomplishments by saying they swam across the English Channel, even though they didn't. -
Potential problems7
AI hallucinations can be a problem for AI systems that are used to make important decisions, such as medical diagnoses or financial trading. -
How to prevent8|9
To prevent AI hallucinations, you can:
– Use data templates to increase the likelihood that the model will generate outputs that align with prescribed guidelines.
– Define boundaries for AI models using filtering tools and/or clear probabilistic thresholds.
– Write prompts that are correct, avoid contradictory aspects, and don't include ambiguous or confusing commentary. -
How AI hallucinations can be useful10
However, AI hallucinations can also be useful in some cases. For example, one team used AI hallucinations to help design a new kind of catheter that greatly reduces bacterial contamination.
K2view on AI hallucinations
To summarize all of the above summaries in one sentence, we define AI hallucinations as the generation of text by large language models that is factually incorrect, nonsensical, and/or ungrounded in its input data.
AI hallucinations are cognitive phenomena, emanating from the patterns your LLM's learned in its training. They innocently appear as well informed answers, but are actually present misleading information, or entirely fabricated content.
02
Understanding AI hallucinations:
The elephant in the china shop
In the ever evolving field of artificial intelligence (AI), Large Language Models (LLMs), like Google Gemini or OpenAI GPT, have demonstrated impressive capabilities in generating natural language text, creating content, coding, and using conversational agents. But there's a major glitch: AI hallucinations.
This article delves into what AI hallucinations are, why they occur, and how the AI community is working to address them.
Generative AI (GenAI) applications are impressive tools capable of generating human-quality text, translating languages, composing music, and much, much more. But a GenAI app can sometimes stumble into the twilight zone, producing spurious or silly responses – known as an AI hallucinations. Such outputs are incorrect, nonsensical, and/or inconsistent.
There are 2 primary reasons why AI hallucinations exist.
The first culprit is the data your LLM was trained on. Just like a student relying on dated textbooks, an LLM trained on a faulty or incomplete dataset will reflect those flaws in its responses. For instance, an AI chatbot trained on a dataset of historical articles filled with factual errors might mistakenly place the Battle of Waterloo in Tahiti.
The second reason is how AI learns. Sometimes, AI models identify patterns in their training data that aren't truly connected – in a process called overfitting. Overfitting occurs when an LLM is trained “too well” on its training data, to the point where it captures the noise and specific details of that data, instead of the underlying distribution. As a result, the LLM performs well on training data but poorly on new data, because it hasn’t yet learned to generalize.
Like a chef who can prepare certain dishes incredibly well but can’t create new ones, the LLM becomes great at regurgitating the training data but can’t innovate, or adapt to new inputs.
The consequences of AI hallucinations can vary in severity. On the lighter side, they might simply be amusing. However, they can also have serious implications, such as an AI-based diagnostic model that misdiagnoses a condition based on the faulty analysis of an x-ray.
Just how prevalent are AI hallucinations?
Vectara continuously evaluates how often leading LLMs introduce hallucinations when summarizing a document. The current findings are stark: Mainstream LLMs are quite hallucinatory, with Google Gemini (1.5) and OpenAI (01) hallucinating more than 3% of the time, with one of the lesser known models (not in the top 25) hallucinating 30% of the time.
Researchers are actively working on solutions to detect and prevent AI hallucinations. One approach involves developing better training methods. By grounding LLMs with higher quality, more diverse data, we can reduce the chances of them learning incorrect information or making spurious connections. In addition to LLM grounding, researchers are creating fact-checking mechanisms that compare GenAI outputs against real-world knowledge bases to identify and flag inconsistencies.
In the corporate world, a GenAI framework called Retrieval-Augmented Generation (RAG) grounds a company’s LLM with structured and unstructured data from its own private sources.
An enterprise LLM augmented with trusted company data reduces AI hallucinations and delivers more meaningful, personalized, and secure responses.
03
What causes AI hallucinations?
Letting the genie out of the bottle
As mentioned above, AI hallucinations stem from the way an LLM learns. The model is trained on vast amounts of data, allowing it to identify patterns and connections between words and concepts. The problem lies in one core LLM function: statistically predicting the most likely word to follow in a sequence. This statistical task can lead LLMs to create content that sounds about right but really isn’t. These deviations can be frustrating and misleading, so understanding the root causes is crucial. Here's a deep dive into the key factors contributing to AI hallucinations:
-
Biases and data noise
LLMs are trained on enormous datasets collected from the internet, which may contain biases or inaccuracies. These imperfections can be reflected in the model's outputs, leading it to hallucinate facts or figures that it wasn't ever taught.
-
Pattern overfitting
LLMs are trained on massive datasets using various statistical methods. During training, the model may memorize specific data patterns to improve short-term accuracy. This practice can lead to overfitting, as discussed in the previous section. Further, statistical analysis may identify correlations between variables that aren't causally connected. An LLM might analyze a an airline’s dataset of ticket purchases and refunds, and mistakenly conclude that all flight tickets purchased online are refundable when, in fact, they’re not.
-
Inherent complexity of natural language
A natural language, like English, is full of context dependencies, idiosyncrasies, and nuances that are difficult for AI to grasp, leading to seemingly irrelevant or ungrounded responses.
-
Lack of real-world understanding
Real-world data is messy and incomplete. If an LLM is trained on a dataset that lacks crucial information or diverse perspectives, it might have trouble representing reality accurately. For example, if an LLM was trained solely on English, it might have difficulty recognizing words like smorgasbord.
-
Issues with common sense
While LLMs excel at pattern recognition and statistical analysis, they lack basic common sense. Imagine the latest RAG conversational AI app tasked with writing a story set in the 1960s. It might generate a scenario where a character uses a cellphone, which, of course, wasn’t invented yet!
-
Evaluating output relevance
Evaluating the quality and factual grounding of AI outputs can be challenging. For example, an employee querying a company chatbot about vacation time won’t find much satisfaction from a response based on publicly available data. And, while a RAG chatbot could provide a generalized response (based on unstructured data in the form of company policy docs), it could only get personal (“You’re entitled to 14.5 vacation days”) when the LLM is grounded with structured data – at the individual employee level.
03
Examples of AI hallucinations:
Phony fabrications
AI, for all its advancements, can sometimes land on Fantasy Island. For example, an AI or RAG hallucination can range from the humorous to the potentially harmful. From misplaced historical events to gravity-defying architecture, here are 10 real-world AI hallucination examples where GenAI got a little carried away, to say the least:
-
Architectural anomalies
An AI-based urban planning app proposed a revolutionary skyscraper design that defied the laws of physics, appearing to float in mid-air. While visually stunning, the design, of course, was structurally impossible.
-
Culinary concoctions
An AI recipe generator proposed a mouthwatering dish featuring an interesting ingredient: "luminescent dragonfruit". The fruit, of course, doesn't exist, but sounds exotic and could have easily tricked someone into trying the recipe regardless. -
Financial fantasies
A stock trading bot, analyzing market trends, recommended investing heavily in a new public company with a revolutionary product – a product, that on further investigation, turned out to be entirely fictitious. -
Historical hiccups
A user asked a GenAI app for a detailed account of the Roman emperor Julius Caesar's assassination. The response wove a thrilling narrative, but mistakenly placed the event in ancient Egypt instead of Rome.
-
Legal liabilities
A legal research AI tool, combing through vast amounts of case law, thankfully threw out a fabricated Supreme Court decision supporting a specific legal argument. The fake case could have misled lawyers and potentially disrupted the legal process. -
Linguistic lapses
An AI-based translator couldn’t figure out a Korean idiom and moved it – as is – into another language, resulting in a flagrantly foul phrase.
-
Lost legends
A GenAI app tasked with writing travel articles described a mystical lost land hidden in the Amazon rainforest, complete with fantastical flora and fauna. The story was captivating but was a complete fabrication based on myths and legends.
-
Musical mishaps
h3>An AI-based music composer tasked with creating a cover in the style of a famous artist generated a nonsensical song that combined elements from completely different genres, a mega musical mixup. -
Scientific sensationalism
A science news chatbot reported on a groundbreaking discovery in physics, claiming scientists achieved perpetual motion – a concept that defies the laws of thermodynamics. The sensational claim grabbed attention but was clearly false.
-
Star scandals
An AI-based tabloid churned out a juicy story about a famous singer's secret marriage to a Martian prince. The outlandish tale spread like wildfire on social media before being taken down (since there are no Martian princes).
04
The consequences of AI hallucinations:
Those "uh-oh" moments
AI hallucinations, while amusing at times, can also result in far-reaching and serious consequences, depending on the context in which they occur. Here's a breakdown of the potential dangers of AI hallucinations:
-
Misinformation dissemination
AI hallucinations can be used to spread misinformation. Consider manipulated social media posts or news articles containing fake news that appears believable. Such articles could easily be used to cause confusion, erode trust in reliable sources, and manipulate public opinion.
-
Wasted resources
In business settings, AI hallucinations can lead to wasted resources based on bad data. For instance, an AI-based marketing tool might recommend a campaign strategy targeting the wrong demographics due to inaccurate, incomplete data.
-
Erosion of trust
Repeated exposure to AI hallucinations can erode trust in the very concept of artificial intelligence. If users get bad info from chatbots or other AI assistants, they probably won’t rely on them for any task.
-
Cyber attacks
In critical applications like self-driving vehicles, AI hallucinations could have life-or-death consequences. Imagine an autonomous taxi thinking one-way street is two-way, as a result of outdated road maps or GPS information .
-
Amplification of bias
AI hallucinations can amplify existing biases present in training data, leading to discriminatory practices if not addressed. For instance, an AI tool used in recruitment might favor candidates from certain backgrounds due to biases in its training data.
05
The upside of AI hallucinations:
The silver lining
While AI hallucinations can be frustrating and misleading, some argue that they also have a positive side in that they can:
-
Spark creativity
Since an AI hallucination often generates unexpected ideas or scenarios, they can challenge us to think outside the box and explore new possibilities.
-
Discover biases in training data
The nonsensical details AI models sometimes make up can be a wake-up call to address these biases and improve the quality of training data.
-
Safeguard against super-intelligence
The unreliability of AI models due to hallucinations prevents them from “taking over the world” – and gives us time to develop safeguards and regulations for AI as it evolves.
-
Ensure job security for real people
The need for a human in the loop due to LLM hallucination issues keeps some jobs relevant that might otherwise be automated. This provides a buffer zone as AI capabilities develop.
While AI researchers should, of course, strive to eliminate hallucinations, we can still pause to appreciate their potential benefits.
06
How to spot AI hallucinations:
If it walks and quacks like a duck...
LLMs are impressive tools, capable of generating human-quality text and completing complex tasks but they're not perfect. AI hallucinations can be convincing, so staying vigilant is crucial. Be on the lookout for:
-
Contextually inconsistent answers
A well-constructed response should flow logically and connect to the information provided. Look for inconsistencies. Does the AI-generated response introduce irrelevant details or veer off-topic? Does it contradict established facts or details mentioned earlier in the conversation? If the answer seems like it belongs in a different story altogether, it might very well be an AI hallucination.
-
Statistically implausible data
LLMs are trained on massive datasets, and sometimes they rely on statistical probabilities to fill in the gaps. This practice can lead to answers that are technically possible but highly unlikely. For example, if you ask an LLM to write a biography of a historical figure, it might include awards or achievements that the person never received. While statistically there's a chance anyone could win any award, the specific details should align with the figure's known history.
-
Emotional manipulation
Be wary of responses that tug at your heartstrings or try to evoke a strong emotional response. While AI can learn to mimic human emotions, it might not understand the nuances of emotional context. An overly sentimental story or a dramatic turn of events could be a sign of fabrication.
-
No references or sources
When providing “known” facts, a reliable AI model should be able to provide some basis for its claims. If you're asking about historical events, scientific discoveries, or specific data points, a legitimate answer should reference credible sources. An LLM that simply states a fact without any source might be hallucinating.
-
Focus on trivia
Although RAG GenAI may be great at mimicking human speech patterns, pay close attention to the content. Does the answer include overly specific or technical details that seem out of place? For instance, an LLM referring to a specific customer refund might add obscure details about the kind of container the goods were shipped in. These overly specific flourishes can be a sign of fabrication.
By recognizing these warning signs, you’ll become a more discerning user of GenAI content. Critical thinking is key to separating fact from fiction in the world of AI.
07
How to prevent AI hallucinations
Researchers are actively exploring several key approaches to create a future where LLMs are more grounded in reality. To eliminate AI hallucinations, AI scientists have their eyes on:
-
Improve training data
The foundation for reliable AI output lies in the data an LLM is trained on. Thus, researchers are prioritizing high-quality data. This involves techniques like data cleaning to remove errors and inconsistencies, and bias filtering to ensure that the LLM learns from a balanced and truthful perspective. The same way a student will perform better when trained on accurate, up-to-date information, the LLM will develop a stronger grasp of reality when fed trusted, fresh data.
-
Check facts
Even the best data can have gaps, so this is where fact-checking mechanisms come into play. As the LLM generates text, these mechanisms compare the output against real-world knowledge bases like scientific publications or verified news articles. For example, consider a RAG LLM responding to a customer query. The fact-checking system would verify details like dates, figures, and locations found in the customer’s private data against publicly available information. Inconsistencies would get flagged, prompting the LLM to revise its response based on verified information.
-
Fine-tune your model
Beyond data cleansing and fact checking, researchers are also focusing on teaching LLMs to reason better. While current RAG AI models excel at pattern recognition and statistical analysis, fine-tuning would allow them to better judge the reliability of their responses. For example, a cellular operator’s chatbot tasked with providing personalized recommendations to users would employ fine-tuning to identify nonsensical elements, like the user in question subscribing to more than one plan at the same time.
-
Strive for transparency
Techniques are being developed to show users the sources the LLM used to generate its response. Sourcing allows users to assess the trustworthiness of the information and identify potential biases in the training data. This transparency allows users to evaluate the information critically and understand the LLM's reasoning process.
-
Activate RAG
A promising approach called Active Retrieval-Augmented Generation (ARAG) provides factual grounding for AI outputs from trusted sources. ARAG uses your private enterprise systems to find relevant information that supplements the LLM's knowledge. This functionality allows the LLM to anchor its responses in real-world data, reducing the risk of fabricated or nonsensical creations.
By combining these approaches, researchers are working towards a future where AI-generated content is grounded. High-quality data, fact-checking, improved reasoning, transparency, and active RAG all play a role in making AI a more reliable and trustworthy resource.
What's keeping you from harnessing the power of GenAI? Watch the video to find out.
08
What the LLMs are saying about hallucinations
Let’s let the LLMs speak for themselves. We asked Google Gemini, OpenAI ChatGPT, and Microsoft Copilot a simple question: "Have you ever provided incorrect information?"
Their responses reveal the fascinating inner workings of AI and the factors that can lead to an AI or RAG hallucination:
Google Gemini
"It's possible that I have unintentionally provided incorrect information in the past. While I am designed to avoid hallucinations, here's why something inaccurate might have slipped through:
-
Data shortcomings: My training data, though vast, might have contained errors or biases. If I rely on that information to answer your query, my response could be misleading.
-
Prompt misinterpretation: Sometimes I might misinterpret your question or miss subtle nuances in your phrasing. This could lead me down the wrong path and generate a response that seems plausible but isn't quite accurate.
-
Statistical flukes: In rare cases, I might generate an answer that appears factually correct based on the statistical patterns in my training data, but is actually incorrect in the real world.
While I can't say for certain if I've ever provided incorrect information due to these factors, I am constantly being improved. Here's what you can do to help:
-
Ask clear questions: The more specific and well-defined your question is, the better I can understand your intent and provide an accurate answer.
-
Fact-check my responses: Don't assume everything I say is true. Double-check the information I provide with reliable sources, especially for critical topics.
-
Provide feedback: If you notice that I've given you incorrect information, let me know! Your feedback helps me learn and improve over time.
By working together, we can ensure that I am a reliable source of information."
OpenAI ChatGPT
"As an AI language model, my responses are generated based on patterns and data from the vast corpus of information I've been trained on. While I don't experience hallucinations or subjective phenomena, there can be instances where the information provided might be inaccurate or misleading due to various factors.
One potential factor is the quality and completeness of the data I've been trained on. While efforts are made to ensure that the training data is diverse and representative, it's impossible to include every possible scenario or piece of information. As a result, there may be gaps or biases in the data that could lead to incorrect responses.
Additionally, the context of the input I receive can influence the accuracy of my responses. If the input is ambiguous or unclear, I might generate a response that doesn't accurately reflect the intended meaning. Similarly, if the input contains incorrect information or is based on faulty assumptions, my response may also be incorrect.
Furthermore, errors in the processing or interpretation of the input can also lead to inaccuracies in my responses. While I strive to interpret and process the input as accurately as possible, there can be instances where mistakes occur, leading to incorrect or nonsensical outputs.
It's essential to approach information provided by any AI with a critical mindset and to cross-reference it with reliable sources to ensure accuracy. If you ever come across any inaccuracies or inconsistencies in my responses, please feel free to point them out, and I'll do my best to provide clarification or correct the information."
Microsoft Copilot
"As an AI language model, I strive to offer accurate and helpful answers based on the vast amount of data and patterns I've learned during my training. However, I am not infallible, and there may be instances where my responses are less accurate or contain errors. These inaccuracies can arise due to various factors:
-
Training data limitations: My knowledge is derived from the text data available during my training, which was up to a certain point in 2021. If new information has emerged since then, I might not be aware of it.
-
Ambiguity and context: Language is inherently ambiguous. Sometimes, context or phrasing can lead to misunderstandings. While I try to infer context, there are cases where I might misinterpret the user's intent.
-
AI hallucinations: Occasionally, I might generate responses that seem plausible but lack factual accuracy. These "hallucinations" occur due to the creative nature of AI models, where they extrapolate from existing data to create new content.
-
Complex topics: Some topics are intricate and multifaceted. Providing concise yet accurate information can be challenging, especially when dealing with scientific, legal, or historical matters.
-
Dynamic world: The world is constantly changing. New discoveries, events, and developments occur daily. Keeping up with real-time information is difficult for any model.
Despite these challenges, I continuously learn and adapt. Feedback from users helps me improve, and I appreciate your understanding. If you ever notice any inaccuracies, please feel free to point them out, and I'll do my best to correct them.
In summary, while I strive for accuracy, I encourage critical thinking and verification from reliable sources. After all, even the most advanced AI models have their limitations, much like the imperfections of human knowledge."
09
Reducing AI hallucinations with GenAI Data Fusion
A great way to reduce AI hallucinations is by using the most advanced RAG tool, one that accesses/supplements both unstructured and structured data from your own private data sources.
This approach, called GenAI Data Fusion, unifies the structured data of a single business entity – customer, employee, or invoice – from enterprise systems based on a data-as-a-product approach.
Data products enable GenAI Data Fusion to access real-time data from multiple enterprise systems, not just static docs from knowledge bases. With this feature, LLMs can leverage RAG to integrate data from your customer 360 platform, and turn it into contextual prompts. The prompts are fed into the LLM together with the user’s query, enabling the LLM to generate a more accurate and personalized response.
K2View’s data product platform lets RAG access data products via API, CDC, messaging, or streaming – in any variation – to unify data from many different source systems. A data product approach can be applied to various RAG use cases to:
-
Resolve problems quicker.
-
Institute hyper-personalized marketing campaigns.
-
Personalize up-/cross-sell suggestions.
-
Identify fraud by detecting suspicious activity in user accounts.
Reduce AI hallucinations with the market-leading
RAG tool – GenAI Data Fusion by K2view.
AI hallucination FAQs
What is an AI hallucination?
According to Google Cloud Topics' AI Hallucinations article, "AI hallucinations are incorrect or misleading results that AI models generate. These errors can be caused by a variety of factors, including insufficient training data, incorrect assumptions made by the model, or biases in the data used to train the model. AI hallucinations can be a problem for AI systems that are used to make important decisions, such as medical diagnoses or financial trading."
How often do AI hallucinations occur?
In a 16 Nov 2023 article, the NY Times reports, "Hallucination rates vary widely among the leading AI companies. OpenAI's technologies had the lowest rate, around 3 percent. Systems from Meta, which owns Facebook and Instagram, hovered around 5 percent."
In a 5 Apr 2024 article, Scientific American explains, "The lowest hallucination rates among tracked AI models are around 3 to 5 percent. To achieve their language fluency, these massive models are trained on orders of magnitude more data than they can store – and data compression is the inevitable result."
In a 26 Jun 2024 article, Medium claims, "The all-too-common myth of GPT 4 having only a 3% hallucination rate is shattered by a recent study that found GPT 4 has a 28.6% hallucination rate. That's almost 10x the oft-cited (i.e., over-hyped) statistic."
How do I stop AI from hallucinating?
In a 15 Feb 2024 article, DigitalOcean writes, "Strategies to mitigate AI hallucinations include using high-quality training data, implementing structured data templates, refining data sets and prompting techniques, and defaulting to human fact-checking for accuracy."
In a 27 Nov 2023 blog, Salesforce advises, "Write more-specific AI prompts. Great generative AI outputs also start with great prompts. And you can learn to write better prompts by following some easy tips. Those include avoiding close-ended questions that produce yes or no answers, which limit the AI's ability to provide more detailed information."
What causes ChatGPT to hallucinate?
University of Arizona Libraries says, "AI tools, like ChatGPT, are trained to predict what words should come next in the conversation you are having with it. They are really good at putting together sentences that sound plausible and realistic. However, these AI models don't understand the meaning behind the words."
Will AI hallucinations ever go away?
In a 19 Nov 2024 blog, InMoment quotes Meta's Yann LeCun admitting, "AI hallucinations are unsolvable."
Writer Barbara Wharton adds, "However, advancements in training and prompt engineering will reduce these occurrences over time. Combining human oversight and good model design practices can help you address hallucinations before they impact your business."
What is the biggest AI hallucination?
In a 1 Oct 2024 blog post, Lettria says, "One of the most common and well-known hallucinations in AI occurs in chatbot systems, particularly those driven by large language models (LLMs). For instance, when an AI-powered chatbot is asked a specific factual question, it may confidently provide a completely wrong answer."