A practical guide to Model Context Protocol (MCP)
What is Model Context Protocol?
Last updated on July 8, 2025
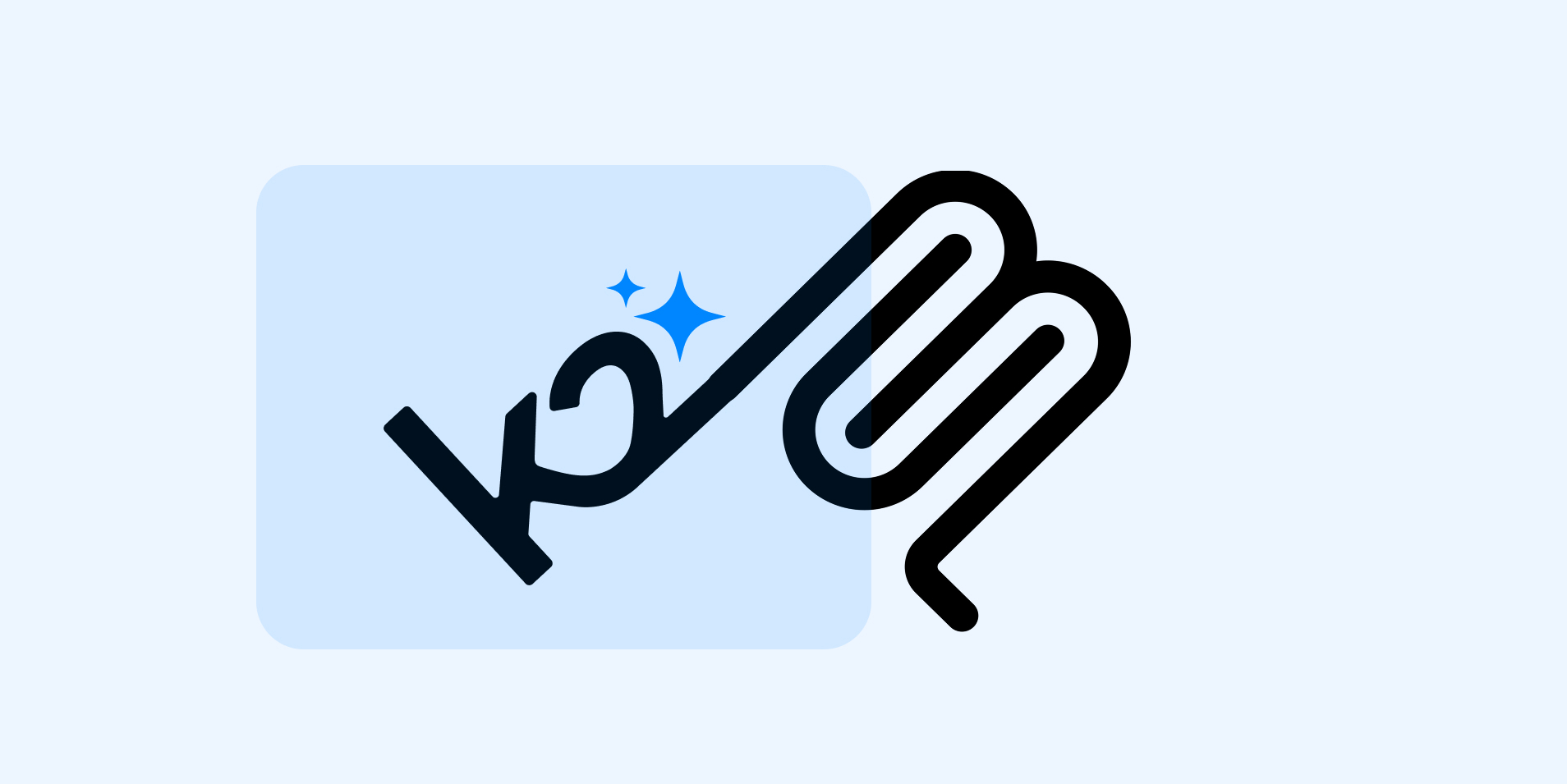
See Agentic AI in Action
Go behind the scenes and see how we ground AI agents with enterprise data
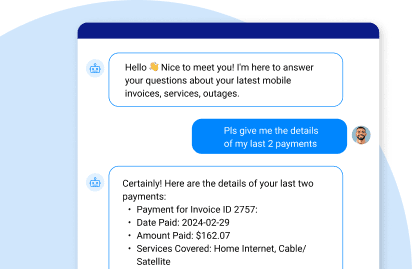
Start your live product tour
Table of Contents
Model Context Protocol (MCP) is an open standard that adds context to queries by enabling LLMs to call external tools and live data sources in real time.
01
What is Model Context Protocol? (MCP)
The Model Context Protocol (MCP) is an open-source protocol developed by Anthropic and released in November of 2024. It represents a significant step forward in enabling easy integration between Large Language Models (LLMs) and a broad range of data sources – addressing the critical need for widespread data access within the realm of generative AI (GenAI).
A protocol defines the rules governing data formatting and processing. MCP LLM establishes a standardized set of rules for how LLMs connect with different external data sources. Such standardization overcomes some of the complexities involved with integrating GenAI with existing enterprise ecosystems.
A recognized standard like MCP AI eliminates the need for customized connectors for every data source. Maintaining context is crucial to generating accurate and relevant LLM responses, so MCP's unified approach to data access is essential for unlocking the full potential of GenAI for the enterprise.1
Anthropic describes model context protocol as “an open protocol that standardizes how applications provide context to LLMs. Think of MCP like a USB-C port for AI applications. Just as USB-C provides a standardized way to connect your devices to various peripherals and accessories, MCP provides a standardized way to connect AI models to different data sources and tools.”2
02
MCP architecture
The model context protocol architecture was specifically designed to enable standardized communication between LLMs and a diverse range of integrations. This section outlines the fundamental components allowing MCP AI to unify data access for generative AI workflows.3
Using Model Context Protocol, one client connects
to as many servers as there are data sources.
Overview
MCP uses a client-server model, in which:
- The MCP client (running inside the host LLM app) opens a direct connection to one or more servers.
- Each MCP server advertises tools, context, and optional prompt snippets.
- When the client calls a tool, the server executes it – pulling data from external sources such as APIs or databases – and streams the result back to the client.
The code for recent interactions with a particular customer might look something like this:
client:
{
"model_id": "chatbot-llm-001",
"entity_type": "customer",
"entity_id": "CUST-39201",
"use_case": "support_summary",
"context_fields": ["recent_interactions", "open_tickets", "account_status"]
}
server response:
{
"entity_type": "customer",
"entity_id": "CUST-39201",
"context": {
"full_name": "Dana Moore",
"account_status": "Active",
"recent_interactions": [
{
"date": "2025-07-01",
"channel": "chat",
"summary": "Customer asked about service outage."
},
{
"date": "2025-07-03",
"channel": "email",
"summary": "Requested refund for overcharge."
}
],
"open_tickets": [
{
"ticket_id": "TCK-11224",
"status": "Pending",
"issue": "Billing discrepancy"
}
]
},
"metadata": {
"retrieved_at": "2025-07-07T14:45:22Z",
"data_quality_score": 0.98
}
}
Core components
-
Protocol layer
The protocol layer handles message framing, request/response linking, and high-level communication patterns. -
Transport layer
The transport layer handles the actual communication between clients and servers. MCP LLM supports multiple transport mechanisms, including Stdio transport for local processes and HTTP with Server-Sent Events (SSE) for server-to-client messages and POST for client-to-server messages. - Message types
MCP supports the following message types:
• Requests expect a response from the other side.
• Results are successful responses to requests.
• Errors indicate that a request failed.
• Notifications are one-way, no-reply messages.
Connection lifecycle
- Initialization
This step establishes the session between the MCP client and server, including authentication, version negotiation, and context setup. It ensures both sides are aligned on protocol rules and ready for message exchange under a shared understanding. - Message exchange
Structured messages are exchanged according to the agreed-upon context. This includes request/response interactions, data transfers, or operational commands, all following strict validation and sequencing rules defined by the protocol. - Termination
Termination cleanly closes the session once communication is complete. - Error handling
This step ensures the system can detect, report, and respond to issues that arise during communication. This includes problems like invalid messages, timeouts, or incompatible versions.
At a high level, MCP is an open protocol that provides a discoverable, composable, and open interface used by GenAI applications to interact with data and tools.
03
Why enterprises adopt the model context protocol
Until MCP came along, connecting LLMs to external data had dev teams creating separate integrations for every API and database – each with different authorizations, data formats, and error handling. By standardizing these interactions MCP delivers4:
1. Quick integrations
With MCP for LLMs, you can plug-‘n-play new capabilities without having to custom-code each from scratch. If there’s an MCP server for a database, then any MCP-compatible LLM can connect to it. The protocol enables LLM funtion calling for retrieving data, querying databases, or recruiting APIs, as needed, just by adding the right server. Imagine a library of pre-made plugins that make specific capabilities available through one standardized protocol.
2. Autonomous agents
MCP let LLM-powered autonomous agents make decisions and perform tasks without human intervention. Autonomous agents use MCP AI to enhance LLM capabilities by integrating with various tools, accessing APIs, retrieving information, and managing workflows in real time. And with memory and reasoning components, they can suggest strategies, learn from past interactions, and continuously improve performance. MCP helps autonomous agents develop not only the thinking – but also the action-taking – of GenAI by giving it standardized access to all relevant data.
3. Easy setup
Because MCP is a universal interface, developers no longer need to maintain separate integrations. Once an application supports MCP, it can connect to any number of services through a single mechanism – reducing the manual setups required each time you want your LLM to use a new API. Dev teams can focus on higher-level logic rather than rewriting connection code for the umteenth time.
4. Universal language
MCP standardizes a universal request-response language across tools so your LLM needn’t cope with one response for one service, and another response for another. All function calls and tool results are communicated in a uniform structure, for simpler debugging and scaling. MCP also ensures your integration logic is future-ready – even if you switch vendors, MCP’s interface to the tools remains the same.
5. Conversational context
MCP maintains context in the never-ending conversation between LLMs and GenAI apps. An MCP server can provide pre-built prompt templates for certain tasks, and plain old data context for others. It allows your LLM to ingest reference data, or follow complicated workflows, without relying solely on APIs. Built to support rich interactions, MCP is especially useful for coding or complex decision making that may require multiple interactions with different data sources.
MCP brings an easily scalable approach to enhancing LLMs, giving them access to the fresh, trusted data they crave while allowing AI agents to tap into knowledge bases, DevOps tools, and enterprise systems.
RE MCP, Gartner predicts that 75% of gateway vendors and 10% of iPaaS providers will have model context protocol features by 2026.
04
Model context protocol use cases
MCP can be applied to a broad range of use cases, notably:
1. Real-time grounding for financial risk
Financial institutions operate in real time to detect fraud, assess risk, and verify identities. With MCPLLM, large language models can access fresh enterprise data to satisfy both customers and compliance laws. They can retrieve transaction, fraud, and customer data from any system for enhanced contextual understanding.
2. Personalized healthcare and patient journeys
Healthcare providers use GenAI to interact with patients on basic tasks like scheduling appointments or sending reminders to update Rx prescriptions. MCP allows secure, compliant streaming of patient histories straight into LLM-powered patient engagement tools while constantly protecting privacy.
3. Customer 360 for retail and telecom
In sectors like retail and telecom, delivering personalized experiences depends on understanding customer context the moment it’s needed. An MPC server provides this context by reviewing order data, interactions, preferences, and service status from multiple underlying systems in real time.
4. Conversational and agentic AI workflows
MCP AI enables conversational and agentic AI workflows to handle complex business operations. For example, LLM-powered autonomous agents may need to issue a support ticket, check regulatory rules, or review delivery status across many systems. MCP empowers agents to decide and act – always in the right context.
5. Compliance, governance, and service automation
In highly regulated industries, all AI-generated answers – and the data that informs them – must be auditable. With MCP, every LLM response can be easily traced back to its data sources. With a single governance layer, enterprises can automate compliance checks, service requests, and reporting.
6. Adoption patterns in the real world
Businesses adopting MCP AI typically start by piloting a single high-value use case, then moving it into production as trust and value are proven. With MCP LLM, models can be enriched with context in minutes for fast time to innovation.
05
MCP best practices
To get the most from a Model Context Protocol implementation, organizations should follow a few key best practices that ensure accurate, secure, and high-performance access to business data for LLM-powered applications.
1. Centralize the MCP server architecture
Rather than deploying an MCP instance for each enterprise system, organizations should adopt a single, unified MCP server that connects to multiple backend systems. A centralized MCP server simplifies integration, reduces operational overhead, and enables consistent context management across use cases. It further acts as the shared brain of enterprise AI, dynamically composing context from distributed systems without duplicating logic or infrastructure.
2. Enforce entity-based data guardrails
AI interactions should be scoped to individual business entities – such as a customer, product, or order – at runtime. Entity-level guardrails restrict LLM access to relevant data only, reducing the risk of exposure, respecting data privacy, and ensuring more focused and accurate responses.
3. Prioritize conversational latency
MCP AI implementations must be optimized for real-time, conversational AI performance. Since the protocol sits on the critical path between user queries and LLM responses, low-latency retrieval of contextual data is essential. Leveraging high-speed data access patterns and memory-resident architectures can significantly reduce response times and improve user experience.
4. Build a rich semantic layer
A well-designed MCP for LLMs should expose a semantic layer that accurately describes each data attribute with business-friendly metadata. This includes data types, relationships, source lineage, and contextual meaning – all of which guide the LLM in forming precise, relevant answers. A rich semantic layer not only improves AI quality but also makes the system more transparent and maintainable.
By following these practices, organizations can ensure their MCP implementation serves as a robust and scalable foundation for AI experiences that are fast, secure, and contextually aware.
06
Model context protocol security risks
While the benefits of MCP can be significant, it also has its security risks5:
1. Stolen tokens and compromised accounts
MCP storage of Open Authorization (OAuth) tokens is a critical vulnerability. For example, if unauthorized users get access to your Gmail token, they’d be able to:
• Access your entire email history
• Send, forward, and delete messages from your account
• Identify and use your Personally Identifiable Information (PII)
2. Compromised MCP Servers
MCP servers represent a particularly attractive target for malicious actors due to their role in centralizing OAuth tokens for many different services. Attackers could:
• Get all your tokens, including those for Gmail, Google Drive, Calendar, and more.
• Take unauthorized actions, across all these interconnected platforms.
• Expose corporate resources, if you linked your work accounts through the MCP server.
• Persist even after you change your password, because OAuth tokens often maintain their validity independently.
3. Indirect prompt injection threats
MCP for AI introduces a new threat through indirect prompt injection. Since the AI assistant interprets natural language commands before sending them on to the MCP server, attackers could craft seemingly benign messages containing concealed malicious instructions.
For instance, an email that appears harmless could contain embedded text that, when processed by the GenAI app, instructs it to forward all financial documents to an external address. This subtle threat is particularly dangerous, as users may be unaware that sharing certain content with their AI could lead to automated and harmful actions being performed through MCP, blurring traditional security boundaries between content viewing and action execution.
4. Lax and aggregated permissions
To provide the broadest possible functionality, MCP servers often request extensive permissions, introducing significant privacy and security concerns, such as the following:
• MCP servers may be granted unnecessarily wide-ranging access to connected services (e.g., full access to your email account instead of more restrictive read-only permissions).
• Centralized storage of authentication tokens might lead to data aggregation in the MCP server.
• Malicious actors who manage to gain access to the server could conduct correlation attacks across interconnected services. For example, with access to both your calendar and email accounts, attackers could mastermind highly targeted phishing or extortion campaigns.
• Legitimate server operators could, in theory, mine aggregated user data across services for commercial gain or to build detailed user profiles.
Additionally, while most apps were originally designed to provide segregated access to user data, the concentration of access to different services within a single protocol may seriously alter established LLM guardrails.
07
MCP challenges in multi-source environments
In addition to the security and privacy risks cited above, MCP faces other challenges, including:
1. Fresh real-time data
Stale data can result in inaccurate suggestions or missed opportunities. A primary challenge for an MCP server is accessing fresh data from the SAP landscape and connected systems. To function effectively, MCP clients require rapid, real-time access to the latest information. Because conversational interactions demand speed, MCP servers must efficiently fetch and process data from multiple sources to ensure timely and relevant responses. Here's a list of the most awesome MCP servers for 2025.
2. Data integration at the speed of AI
Retrieving information for AI agents about customers, suppliers, employees, or other business entities entails integrating data from multiple systems like SAP, Salesforce, Workday, and support platforms. Each of these systems would require its own MCP server, delegating cross-system data harmonization to the AI agent. This implies that agentic AI systems must be supported by:
• Metadata enrichment and semantic layers
• Entity resolution (master data management)
• Tooling descriptions and ontology mappings
• Aggregator layers that unify responses
• Few-shot models (chain-of-thought reasoning)
3. Precise responses
Without current and unified data access, LLMs may generate plausible but incorrect information based on incomplete or outdated data.
To address these challenges, generative AI techniques such as chain-of-thought prompting (guiding the model step by step), retrieval-augmented generation (retrieving data at runtime), and table-augmented generation (querying and interpreting tabular business data) need to be implemented.
Additionally, metadata enrichment and management (data cataloging), data governance (data quality and privacy enforcement), and real-time data integration are required.
Solving these data access hurdles is crucial for organizations aiming to leverage the full capabilities of AI agents, grounded in accurate and secure business information.
These challenges were highlighted in a recent K2view survey, where fragmented, hard-to-access data was identified as a significant obstacle by most respondents.
08
RAG vs MCP
Model Context Protocol is not the first attempt at standardizing context injection to LLMs. Other frameworks, like Retrieval-Augmented Generation (RAG), also ground LLMs with internal data. What’s the difference between these approaches?
While both RAG and MCP deliver context to GenAI apps, they can be seen as complementary or potentially overlapping approaches to enhancing the accuracy and security of LLM responses within the context of enterprise data. Let’s take a closer look at their relationship.
Attribute | Retrieval-Augmented Generation (RAG) | Model Context Protocol (MCP) |
Objective | To ground LLMs on enterprise data | To connect GenAI apps to external data |
Procedure | Retrieves relevant data at query time | Defines a protocol based on a client-server model |
Focus | Provides LLMs with the context to answer accurately | Accesses and interacts with data in a governed manner |
Data access | Fetches data based on the semantic similarity of a query | Controls data movement between client and server |
Context injection | Injects context into the prompt | Injects context via the data retrieved from the MCP servers |
Hallucination prevention | Delivers specific trusted data | Ensures that data is drawn from authorized sources |
Real-time data | Works with real-time data sources | Streams enterprise data in real time |
Security and governance | Adds security to data retrieval | Has MCP guardrails built in |
Use cases | Customer service chatbots and AI virtual assistants | Agentic AI models that are trained to decide and act |
In theory, RAG could be implemented in an MCP infrastructure. A GenAI app (MCP client) could use the protocol to query an MCP server for relevant data. The MCP server, in turn, could orchestrate data retrieval from various sources, potentially including enterprise systems and knowledge bases used for RAG-style retrieval. The retrieved information could then be used as context for the LLM's generation.
MCP and RAG are not mutually exclusive. MCP can provide the secure and governed data access layer that RAG can then leverage to retrieve specific context for its generation process. MCP offers a broader framework for AI-data interaction, while RAG is a specific technique focused on improving the quality of generated text based on retrieved information.
09
Adopt a data product approach with MCP
GenAI Data Fusion, the RAG tool by K2view, acts as a single MCP server for any enterprise. Instead of building unique integrations for each LLM or AI project, every data product, whether sourced from the cloud or from legacy systems, is discoverable and served through the MCP protocol – bringing true business context and scale to your GenAI apps.
K2view is unique in its ability to work with both structured and unstructured data. MCP ensures that the K2view platform serves only the most current, relevant, and protected data to LLMs and agentic AI workflows.
GenAI Data Fusion delivers:
- Unification of fragmented data
Data is aggregated from all sources and exposed at conversational latency for immediate use. - Granular privacy controls
PII and other sensitive is always protected, because only authorized users and use cases can access it. - Real-time data delivery to AI agents and LLMS
Data is delivered via built-in data virtualization and transformation capabilities for consistency and context. - Support for on-prem and cloud environments
Enterprises can deploy their GenAI tools anywhere they want. - Complete auditability
Each context package can be traced, and every access is logged for compliance.
These features are essential for regulated industries, and for any enterprise where fresh answers and trustworthiness are required.
Survey data from our State of Data for GenAI report shows only 2% of businesses are currently ready for GenAI at scale, the biggest barriers being the inability to access fragmented data, poor lineage, and privacy gaps.
With MCP, the K2view platform overcomes all of these challenges.
10
Conclusion
Integrating data for AI agents from multiple systems – via SAP MCP, Salesforce MCP, and various others – presents significant challenges. Each source often requires its own MCP server, leaving the complex work of metadata enrichment, entity resolution, privacy enforcement, and real-time access to the LLM agents themselves.
Fragmented data, inconsistent governance, and the risk of stale or incomplete information further increase complexity and the possibility of errors, making it difficult to provide AI agents with timely, coherent, accurate, and secure data.
With K2view, one client connects
to one server for all data sources.
K2view GenAI Data Fusion overcomes these challenges by acting as a single, unified MCP server that connects, enriches, and harmonizes data from all core systems.
Its patented semantic data layer makes both structured and unstructured enterprise data instantly and securely accessible to GenAI apps through one MCP server, ensuring real-time, unified information for accurate and personalized AI responses across the enterprise.
Model Context Protocol FAQ
What is Model Context Protocol (MCP)?
MCP is an open protocol that standardizes how applications provide context to LLMs. Think of MCP as the central bus station for AI apps. Just as the station provides one venue for all connections, MCP provides a standardized way to connect AI models to different data sources and tools.6
What is the use of MCP?
MCP is designed primarily for developers building custom integrations and AI applications. MCP enterprise deployments are ideal for teams with technical resources that need to build specialized AI capabilities into their own applications or workflows.7
What is an LLM MCP?
The Model Context Protocol (MCP) is set to be the standard for connecting LLM applications to external data sources and tools. Introduced by Anthropic in November, it has since gained broad backing, including from OpenAI, Microsoft, and Google.8
What is MCP in AI agents?
Model Context Protocol (MCP) is an open standard developed by Anthropic, the company behind Claude. While it may sound technical, but the core idea is simple: give AI agents a consistent way to connect with tools, services, and data — no matter where they live or how they're built.9
Is Model Context Protocol free?
The Model Context Protocol is an open-source project run by Anthropic, PBC. and open to contributions from the entire community.10
What are MCP tools?
MCP tools allow servers (an MCP SQL server, for example) to expose executable functions that can be invoked by clients and used by LLMs to perform actions. Key aspects of tools include:11
Why do we need MCP?
MCP is a fundamental shift that could reshape how we build software and use AI. For AI agents, MCP is transformative because it dramatically expands their reach while simplifying their design. Instead of hardcoding capabilities, an AI agent can now dynamically discover and use new tools via MCP.12
Why do we use MCP?
MCP servers can expose various tools and resources to AI models, enabling functionalities such as querying databases, initiating Docker containers, or interacting with messaging platforms like Slack or Discord.13
What is the MCP protocol?
The Model Context Protocol (MCP) is an open standard introduced by Anthropic with the goal to standardize how AI applications (chatbots, IDE assistants, or custom agents) connect with external tools, data sources, and systems.14
What problem does MCP solve?
Every new data source requires its own custom implementation, making truly connected systems difficult to scale. MCP addresses this challenge by providing a universal, open standard for connecting AI systems with data sources, replacing fragmented integrations with a single protocol.15