Table of Contents
Table Augmented Generation (TAG) is a framework that improves the accuracy of GenAI responses by injecting structured enterprise data into the LLM prompts.
Automatically responding with trustworthy answers to user queries, particularly when relying on an organization's private, confidential data, continues to be a challenge for businesses seeking to leverage the potential of their Generative AI (GenAI) applications. While technologies like Machine Translation and Abstractive Summarization can facilitate communication and create engaging interactions, the goal of consistently generating precise, dependable responses remains elusive.
01
What is table-augmented generation?
Table-Augmented Generation (TAG) is an approach within Generative AI (GenAI) that enhances Large Language Models (LLMs) by integrating up-to-date, structured data straight from your enterprise systems, leading to more accurate and personalized outputs.
The term "Table-Augmented Generation (TAG)" derives from "Retrieval-Augmented Generation (RAG)", an unstructured data retrieval process described in a 2020 paper from Facebook AI Research (now Meta AI), which outlined a method for improving knowledge-intensive tasks. The publication presented the idea of a "general-purpose refinement strategy" designed to link LLMs with various internal or external data sources.
Essentially, it incorporates a data extraction step into the output creation process, aiming to improve the pertinence and dependability of the generated responses.
The data extraction mechanism identifies, selects, and prioritizes the most pertinent documents and information from suitable sources based on the user's inquiry. This information is then transformed into an enhanced, context-rich prompt, which is then sent to the LLM via its API. The LLM then produces a precise and logical response for the user.
A useful analogy for TAG involves a stock trader who combines publicly available historical financial data with real-time market updates.
Traders within a financial firm make investment decisions by analyzing market trends, industry developments, and company performance. However, to maximize profitable trades, they also utilize live market data and internal stock recommendations provided by their firm.
In this scenario, the publicly available financial data mirrors the LLM's pre-existing knowledge, while the live data feeds and the firm's internal recommendations represent the internal sources utilized by the TAG system.
02
Getting it right ain't easy
Conventional text generation models, often built on encoder/decoder structures, possess capabilities for language translation, stylistic variations in responses, and answering straightforward inquiries. However, these models operate based on statistical patterns derived from their training datasets, which can occasionally lead to the generation of inaccurate or irrelevant information, often referred to as hallucinations.
Generative AI (GenAI) utilizes Large Language Models (LLMs) trained on extensive volumes of publicly accessible (Internet) data. The resource-intensive nature of retraining these models, in terms of both cost and time, limits the frequency with which LLM providers (such as Microsoft, Google, AWS, and Meta) can update them. Therefore, LLMs, which incorporate public data up to a specific point in time, lack access to the most current information and the valuable private data locked inside your organization. This inherent limitation contributes to the problem of AI hallucinations.
Table-Augmented Generation (TAG) is a developing GenAI technique that addresses these shortcomings.
A comprehensive TAG deployment involves the retrieval of structured data from enterprise systems and unstructured data from internal knowledge bases.
By converting real-time business data into intelligent, context-sensitive, and compliant prompts, TAG prevents hallucinations and enhances the overall reliability of GenAI apps.
In its 2024 Generative AI report, Gartner advises IT, data, and analytics executives wishing to integrate GenAI with private and public corporate data to prioritize investments in augmented generation.
03
TAG architecture
The following diagram shows a table-augmented generation framework and data flow, from user prompt to LLM response:
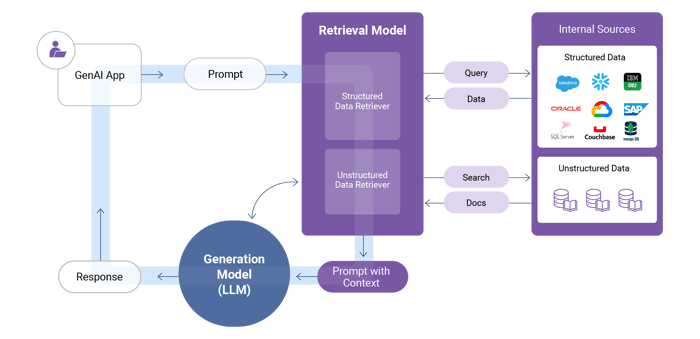
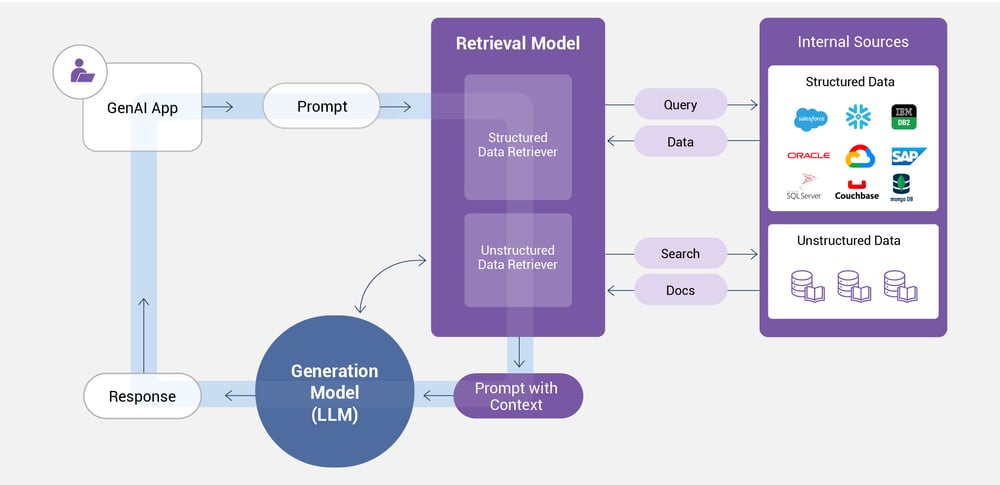
-
A user asks a question, prompting the data retrieval model to access your company's internal data as needed.
-
The retrieval model searches the your internal data stores for structured data from your enterprise systems.
-
The retrieval model crafts an enriched prompt – which augments the user's original query with supplementary contextual details, and forwards it on, as input to the generation model (LLM).
-
The LLM uses the augmented prompt to produce a more precise and pertinent response, which is then presented to the user.
The query-to-response process should only take a couple of seconds to mimic a natural conversation.
04
Table-augmented generation challenges
Producing precise, dependable, and relevant responses is crucial.
However, TAG also presents certain challenges:
- Enterprise TAG depends on data retrieval across the entire organization. It's essential not only to maintain current and accurate data but also to have precise metadata that accurately describes your data for the TAG framework.
- The data stored in your enterprise systems must meet the data quality for AI standards of compliance, completeness, and freshness. For instance, if you manage 100 million customer records, can you retrieve and integrate all the data relevant to David Smith in under a second?
- Generating insightful contextual prompts requires advanced AI prompt engineering skills, such as chain-of-thought reasoning, to effectively inject the relevant data into the LLM in a manner that yields the most accurate responses.
- To safeguard data privacy and security, LLM access must be restricted to authorized data only. Using the prior example, this means providing access solely to David Smith's data and to no one else's.
In addition to these challenges, organizations have expressed further concerns, as highlighted in our recent survey below:
Top concerns in leveraging enterprise data for GenAI and RAG
Source: K2view Enterprise Data Readiness for GenAI in 2024 report
Given the equal distribution of key concerns, it's clear that focusing solely on one of them – such as scalability and performance, while neglecting data quality and consistency, real-time data integration and access, data governance and compliance, or data security and privacy – will not suffice. To effectively combine enterprise data with GenAI initiatives, a comprehensive, integrated strategy is needed.
"Organizations are continuing to make significant investments in GenAI," says the 2024 Gartner LLM report, Lessons from Generative AI Early Adopters. "But they encounter difficulties related to technical implementation, expenses, and talent acquisition."
Despite these challenges and concerns, TAG represents a significant advancement in GenAI technology. Its capacity to leverage fresh enterprise data addresses the limitations of traditional generative models by enhancing user interactions with more personalized and dependable responses.
TAG is already proving its worth in various sectors, including customer support, IT service management, sales and marketing, and legal and compliance. But the optimal GenAI approach – whether TAG or prompt engineering vs fine-tuning – must be assessed based on the use case at hand.
05
Table-augmented generation use cases
Enterprises use TAG for multiple domains, including:
Department |
Objective |
Types of RAG data |
Customer service |
Customize chatbot responses – based on a customer’s behaviors, needs, preferences, and status – to respond in a more personalized way. |
|
Sales and marketing |
Interact with customers via chatbot or virtual assistant to describe products and offer tailored recommendations. |
|
Compliance |
Respond to customers requests. |
|
Risk |
Identify fraud. |
|
06
Benefits of table-augmented generation
In the realm of generative AI, your data serves as a key competitive advantage. Implementing TAG offers several benefits:
- Accelerated value realization at reduced expense
Training an LLM demands significant time and resources. By providing a faster and more cost-effective method for incorporating new data into the LLM, TAG makes generative AI both accessible and dependable for both customer-facing and internal operations. - Tailored user interactions
By combining detailed customer 360 data with the broad general knowledge of the LLM, TAG enables personalized user interactions through chatbots and customer service representatives, delivering real-time, customized recommendations for next-best-actions, cross-selling, and upselling. This allows for scalable AI personalization. - Enhanced user confidence
TAG-powered LLMs deliver credible information by ensuring data accuracy, up-to-dateness, and relevance, all tailored to the individual user. This fosters user trust, which in turn safeguards and enhances your brand's reputation. - Better user experiences and lower customer support costs
Chatbots powered by customer data enhance the user experience and drive down customer care expenses by boosting first-contact resolution rates and reducing the overall volume of service calls.
07
TAG deployment tips
In its December 2023 report, Emerging Tech Impact Radar: Conversational Artificial Intelligence, Gartner projects that widespread organizational adoption of TAG will require several years due to the intricate nature of:
- Integrating GenAI into self-service customer support systems, such as chatbots.
- Ensuring the confidentiality of sensitive data by restricting access to unauthorized individuals.
- Merging insight engines with knowledge repositories to facilitate search retrieval functionalities.
- Indexing, embedding, preprocessing, and/or visualizing enterprise data and documents.
- Developing and integrating retrieval pipelines into applications.
These challenges are compounded by skill deficiencies, data dispersion, ownership disputes, and technological limitations within enterprises.
Furthermore, as vendors introduce tools and workflows for data onboarding, knowledge base activation, and components for TAG application design (including conversational AI chatbots), organizations will actively engage in data grounding for content consumption.
In the January 2024 Gartner TAG report, Quick Answer: How to Supplement Large Language Models with Internal Data, Gartner GenAI analysts recommend that enterprises preparing for TAG:
- Choose a pilot use case where business value can be clearly quantified.
- Categorize your use case data as structured, semi-structured, or unstructured to determine optimal data handling and risk mitigation strategies.
- Compile comprehensive metadata, as it provides context for your TAG deployment and informs the selection of enabling technologies.
In its January 2024 article, Architects: Jump into Generative AI, Forrester asserts that for TAG-centric architectures, gatekeeping mechanisms, pipelines, and service layers are most effective. Therefore, when considering the implementation of GenAI applications, make sure that they:
- Include intent and governance controls at both entry and exit points.
- Use pipelines capable of engineering and governing prompts.
- Address LLM grounding through TAG.
08
Structured data retrieval
The TAG life cycle – from data augmentation to generation – is based on a Large Language Model or LLM.
An LLM is a fundamental Machine Learning (ML) model that utilizes deep learning algorithms for natural language processing. It is trained on vast quantities of publicly available text data to comprehend complex language patterns and relationships, enabling it to perform tasks such as text generation, summarization, translation, and question answering.
These models are pre-trained on extensive and diverse datasets to master the nuances of language processing and can be further refined for specific applications or tasks. The term "extensive" barely captures the scale, as these models can encompass billions of data points. For instance, GPT 4.o is reported to contain over a trillion.
TAG implements retrieval and generative models through 4 key stages:
- Data access
The initial step in any TAG system involves data access. The retrieval model queries your enterprise data to identify and gather relevant information. To ensure accurate, diverse, and reliable data sourcing, it's crucial to maintain precise and current metadata, as well as manage and minimize data redundancy. - Preparing enterprise data for retrieval
Companies must organize their multi-source data and metadata to enable real-time access by TAG. For example, customer data, including master data (unique customer identifiers), transactional data (e.g., service requests, purchases, payments, and invoices), and interaction data (emails, chats, phone call transcripts), must be integrated, unified, and organized for immediate retrieval. Depending on the use case, data may need to be arranged by other business entities, such as employees, products, suppliers, or any other relevant factor. - Data protection
The TAG framework must implement role-based access controls to prevent users from accessing information beyond their authorized scope. For example, a customer service representative dealing with a specific customer should be restricted from accessing data related to other customers, and a marketing analyst should not have access to certain confidential financial data. And any sensitive data retrieved by TAG, such as Personally Identifiable Information (PII), must be protected from unauthorized access, like credit card details for salespeople or Social Security Numbers for service agents. The TAG solution must employ dynamic data masking to ensure compliance with data privacy regulations. - Prompt engineering for enterprise data
To properly integrate enterprise data, the table-augmented generation process constructs an enriched prompt by synthesizing a "story" from the retrieved 360-degree data. Continuous prompt engineering tuning, ideally supported by Machine Learning (ML) models and advanced chain-of-thought prompting techniques, is essential. For instance, the LLM can be asked to reflect on its existing data and identify necessary additional data or explain why a user terminated a conversation.
09
Real-time data retrieval
The need for TAG to access enterprise application data in real time can clearly be seen in customer service chatbot use cases, where immediate responses are required.
The data needed for generative AI use cases in customer service is typically found in CRM, billing, and ticketing systems.
A real-time, comprehensive understanding of the relevant business entity, be they customers, employees, suppliers, products, or loans, is essential.
However, the procedure for locating, accessing, integrating, and unifying real-time enterprise data, and then integrating it with your LLM, is very intricate due to:
- Fragmented enterprise data dispersed among many different systems, each with its distinct databases, structures, and formats.
- High complexity and cost of data management required for real-time retrieval of the data pertaining to a single entity (such as an individual customer).
- Performance issues arising from high-volume and low-latency demands.
- Consistently delivery of clean, complete, and up-to-date data.
- Privacy and security mandates that limit access to authorized data only.
- AI database schema generator for cross-system data visibility.
10
TAG in its current state
Numerous organizations are currently testing TAG chatbots with internal users, such as customer service agents, as they remain cautious about deploying unsupervised systems in production environments. These concerns are centered around generative AI hallucinations, privacy, and security. As GenAI systems demonstrate increased reliability, this trend is expected to shift.
TAG apps are rapidly emerging across various sectors. Examples might include:
-
Car owners requesting claim histories to reduce annual premiums
-
Hospital patients wanting to cross-reference the treatments they received to the payments they made
-
Stock investors wishing to see the commissions they were charged year over year
-
Telco subscribers selecting new plans since theirs are about to terminate
TAG is currently being used to deliver precise, context-aware, and up-to-date answers to inquiries through chatbots, email, text messaging, and other conversational AI applications. In the future, TAG might propose suitable actions based on contextual information and user prompts.
For example, while today GenAI might be used to provide army veterans with information about higher education reimbursement policies, it could eventually list nearby colleges and even recommend programs tailored to the applicant's prior experience and military training. It might even be capable of automatically generating the reimbursement request itself.
11
Data readiness for GenAI
At the end of the day, it’s hard to deliver strategic value from GenAI today because your LLM lacks business context, costs too much to retrain, and hallucinates too frequently. What's needed is LLM grounding on a consistent basis.
K2view GenAI Data Fusion is a patented table-augmented generation solution that grounds your generative AI apps with real-time enterprise data from any source.
With GenAI Data Fusion, your data is:
- Ready for GenAI
- Deliverable in real time and at enterprise scale
- Complete and fresh
- Compliant and trusted
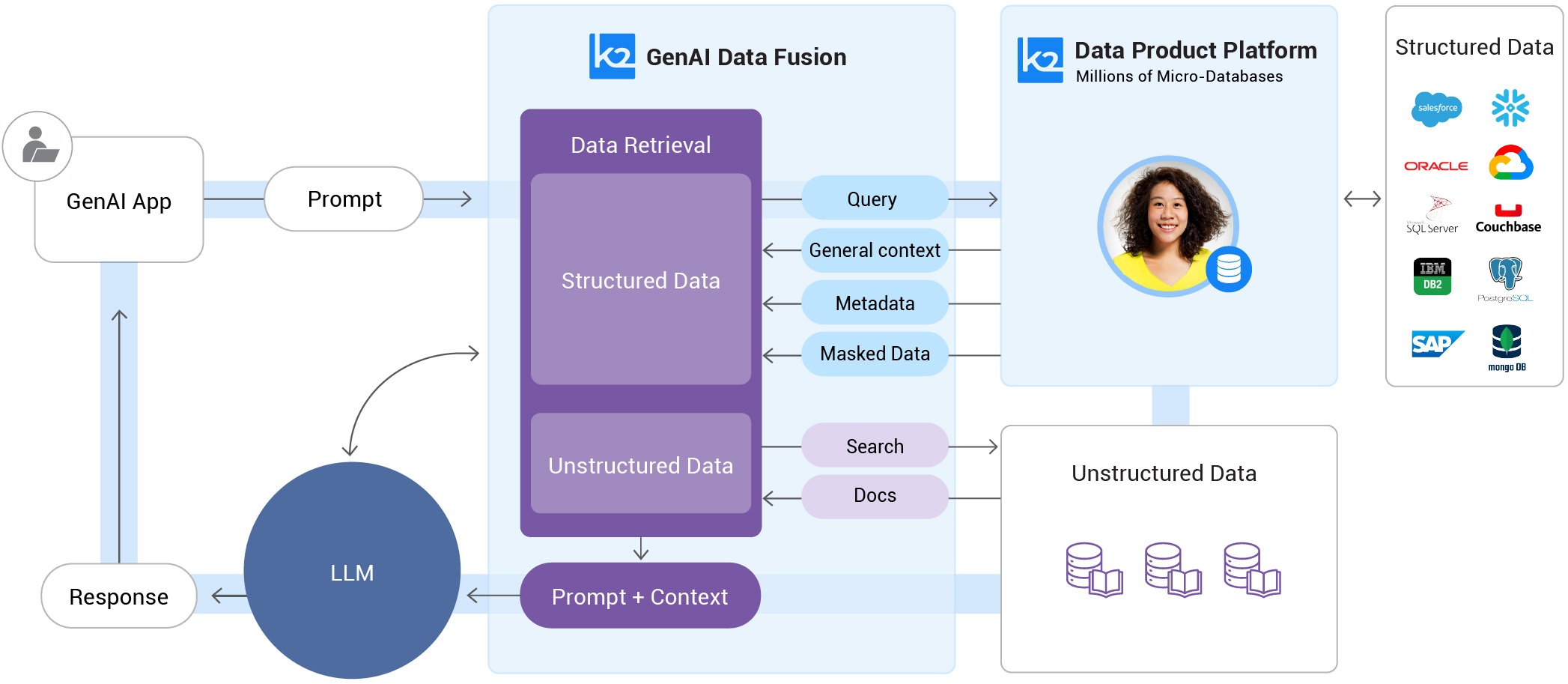
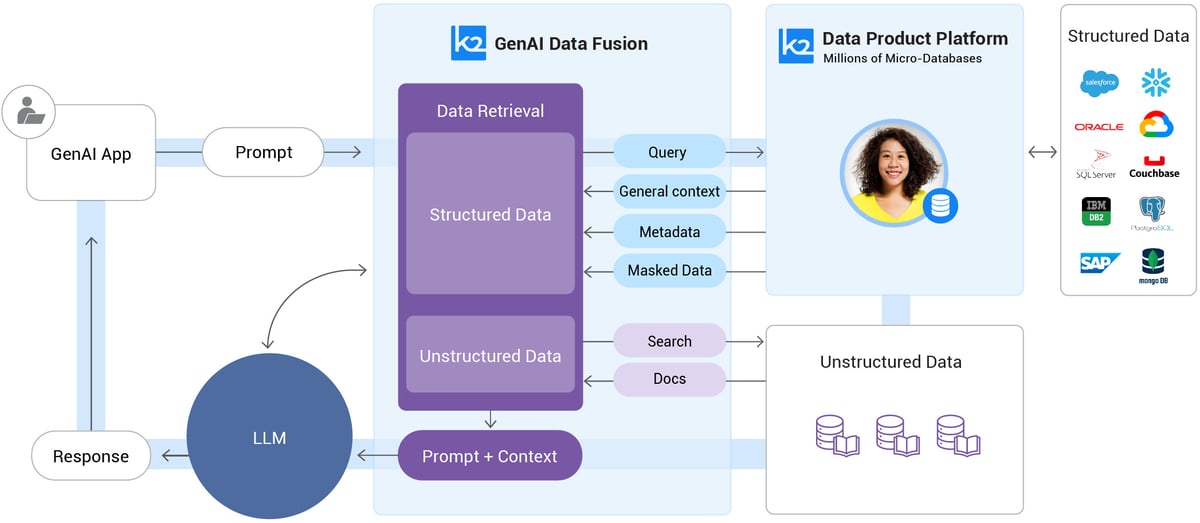
The diagram shows how the K2view Data Product Platform and Micro-Database™ technology enable GenAI Data Fusion to access structured data:
-
The user enters a prompt, triggering GenAI Data Fusion to access your structured enterprise data.
-
Micro-Database technology queries only the relevant business entity (e.g., specific customer).
-
The original prompt is augmented with the entity's data, metadata, and context to create intelligent prompts for the LLM.
-
The LLM uses the augmented prompt to generate a personalized and reliable response to the user.
Supercharging TAG with enterprise data
K2view GenAI Data Fusion organizes your fragmented enterprise data into 360° views of your business entities, making it instantly accessible.
Each 360° view is stored in its own secure Micro-Database, 90% compressed and built for low-footprint, low-latency TAG access.
K2view organizes the data for each business entity in its own high-performance Micro-Database – always ready for real-time access by TAG workflows.
By continually syncing with underlying sources, Micro-Databases can be modeled to capture any business entity – and comes with built-in data access controls and dynamic data masking capabilities.
Micro-Database technology features:
-
Real-time data access, for any business entity
-
360° views of any entity
-
Fresh data all the time, in sync with underlying systems
-
Role-based access controls (RBAC), to maintain data privacy
-
Lower TCO, thanks to a small footprint and the use of commodity hardware
An entity’s data can be queried by, and injected into, your LLM as a contextual prompt in milliseconds. Entity-based TAG assures AI data readiness.
GenAI Data Fusion can:
-
Retrieve and augment structured data about a customer or any other entity.
-
Dynamically mask PII (Personally Identifiable Information) or other sensitive data.
-
Be reused to handle data service access requests, or suggest cross-sell recommendations.
-
Access enterprise systems via API, CDC, messaging, streaming – in any combination – to aggregate data from multiple source systems.
It powers your GenAI apps to respond with accurate recommendations, information, and content – and without LLM hallucination issues – for a wide range of use cases, such as:
Speeding up |
Coming up with |
Suggesting |
Detecting |
Learn more about the K2view GenAI Data Fusion TAG tool.
Table-Augmented Generation FAQs
1. What is Table-Augmented Generation (TAG)?
Table Augmented Generation (TAG) is an AI technique that combines the strengths of machine learning with database knowledge. It uses a table-based structure to generate results that are not only accurate but also contextually relevant. TAG’s primary goal is to augment the outputs from Text2SQL, which translates natural language queries into SQL commands, by using AI-powered insights to refine and improve these results.1
2. How is TAG different from RAG and GAG?
TAG (Table-Augmented Generation) shares similarities with both RAG (Retrieval-Augmented Generation) and GAG (Graph-Augmented Generation), but distinct differences set it apart:1
-
RAG (Retrieval-Augmented Generation)
RAG combines retrieval-based methods with generative models to enhance the quality of generated text by incorporating relevant information from external documents(structured/unstructured). It is primarily used in contexts where the generation of text needs to be informed by specific, retrieved content.
-
GAG (Graph-Augmented Generation)
GAG emphasizes the integration of graph-based data structures with machine learning to generate more accurate and informative outputs. GAG’s primary focus is on leveraging graph data.
-
TAG (Table Augmented Generation)
TAG specifically integrates database querying (via Text2SQL) with AI-driven augmentation. It is designed to work with structured data in databases, making it particularly useful for data analysis and business intelligence applications.
3. How does TAG work?
Below are the steps involved in table-augmented generation:2
-
Table extraction
In this step, when a user provides a query or prompt (for example, “Generate a report on sales performance for Q1”), the system identifies relevant structured data sources, such as tables from databases or spreadsheets. The system analyzes the content of these tables to find specific rows, columns, or entries that are relevant to the user’s request. This could include sales figures, customer data, or product information from a financial or business report table.
-
Data fusion
Once the relevant table or data from structured sources is extracted, the next step is to combine this data with a generative model, such as GPT. Here, the model takes the raw data from the table (numbers, facts, and relationships between different fields) and integrates it into the generative process. The model doesn’t just list the numbers but uses them to generate coherent text that explains or interprets the table’s data, such as “Sales increased by 15% in Q1 compared to Q4, driven by a rise in product X sales.”
-
Output generation
The final step is where the generative model produces fluent and coherent text based on the structured data. The output could be a summary, a report, or any content that reflects the relationships and values present in the table. The model ensures that the generated content aligns accurately with the original structured data, maintaining consistency in terms of the numbers and insights derived from the table. This guarantees that the final text not only makes sense but is also grounded in the actual data from the extracted tables.
In this process, the AI can generate highly accurate and fact-based content, making it ideal for applications like financial reporting, product summaries, or automated business analysis where precise numerical and structured data need to be incorporated into natural language text.
4. What are TAG's key components?
There are the 5 key components in a table-augmented generation framework:2
-
Table parser
This component is responsible for identifying and extracting relevant structured data from tables, spreadsheets, or databases based on the user’s query or prompt. It analyzes the table’s structure (rows, columns, headers) to extract useful information that aligns with the input request.
-
Data integration/fusion
After extracting the relevant table data, this component integrates the structured data with the generative model. The data is embedded into the model’s generation process, allowing the AI to incorporate facts, figures, and relationships from the table into the generated text.
-
Generator
This is the core generative model (e.g., GPT, BERT) that produces human-readable text. The generator synthesizes the extracted table data and creates output that reflects the structure and information from the table, ensuring the generated text is coherent, contextually relevant, and factually accurate.
-
Table retrieval system
In cases where there are multiple tables or data sources, this system retrieves the most relevant tables or structured data to the prompt. It decides which tables or portions of structured data will be used to generate the desired content.
-
Post-processing/validation
This component ensures that the generated text aligns correctly with the structured data and that the factual accuracy of the output matches the original data. It may involve verifying numbers, dates, and relationships from the table to prevent errors in the final content.
These components work together in TAG to ensure that structured data from tables is accurately and seamlessly incorporated into the text generation process, making it ideal for applications like report writing, data summarization, or any content where precision is crucial.
5. What are TAG's key benefits?
TAG offers several key advantages:3
-
Improved accuracy
By directly using structured data, TAG minimizes hallucinations and ensures more accurate outputs compared to models relying solely on unstructured text.
-
Real-time data integration
TAG allows for the incorporation of real-time data from dynamic tables, enabling up-to-date insights.
-
Enhanced context retention
Utilizing HTML structures like tables helps retain context and reduce noise, leading to more coherent and relevant generated content.
-
Suitability for specific industries
TAG is particularly beneficial in sectors like finance, retail, and healthcare, where structured data is fundamental for decision-making and reporting.
6. How does TAG combine AI with databases?
AI systems that serve natural language questions over databases promise to unlock tremendous value. Such systems would allow users to leverage the powerful reasoning and knowledge capabilities of Large Language Models (LLMs) alongside the scalable computational power of data management systems.
These combined capabilities would empower users to ask arbitrary natural language questions over custom data sources. However, existing methods and benchmarks insufficiently explore this setting.
-
Text2SQL
Text-to-SQL methods focus solely on natural language questions that can be expressed in relational algebra, representing a small subset of the questions real users wish to ask.
-
Retrieval-Augmented Generation (RAG)
RAG considers the limited subset of queries that can be answered with point lookups to one or a few data records within the database.
-
Table-Augmented Generation (TAG)
TAG is a unified, general-purpose paradigm for answering natural language questions using databases.
The TAG model represents a wide range of interactions between the LLM and database that have been previously unexplored and creates exciting research opportunities for leveraging the world knowledge and reasoning capabilities of LLMs over data.3